Integration Best Practices: Why You Should Limit Integration Run Times
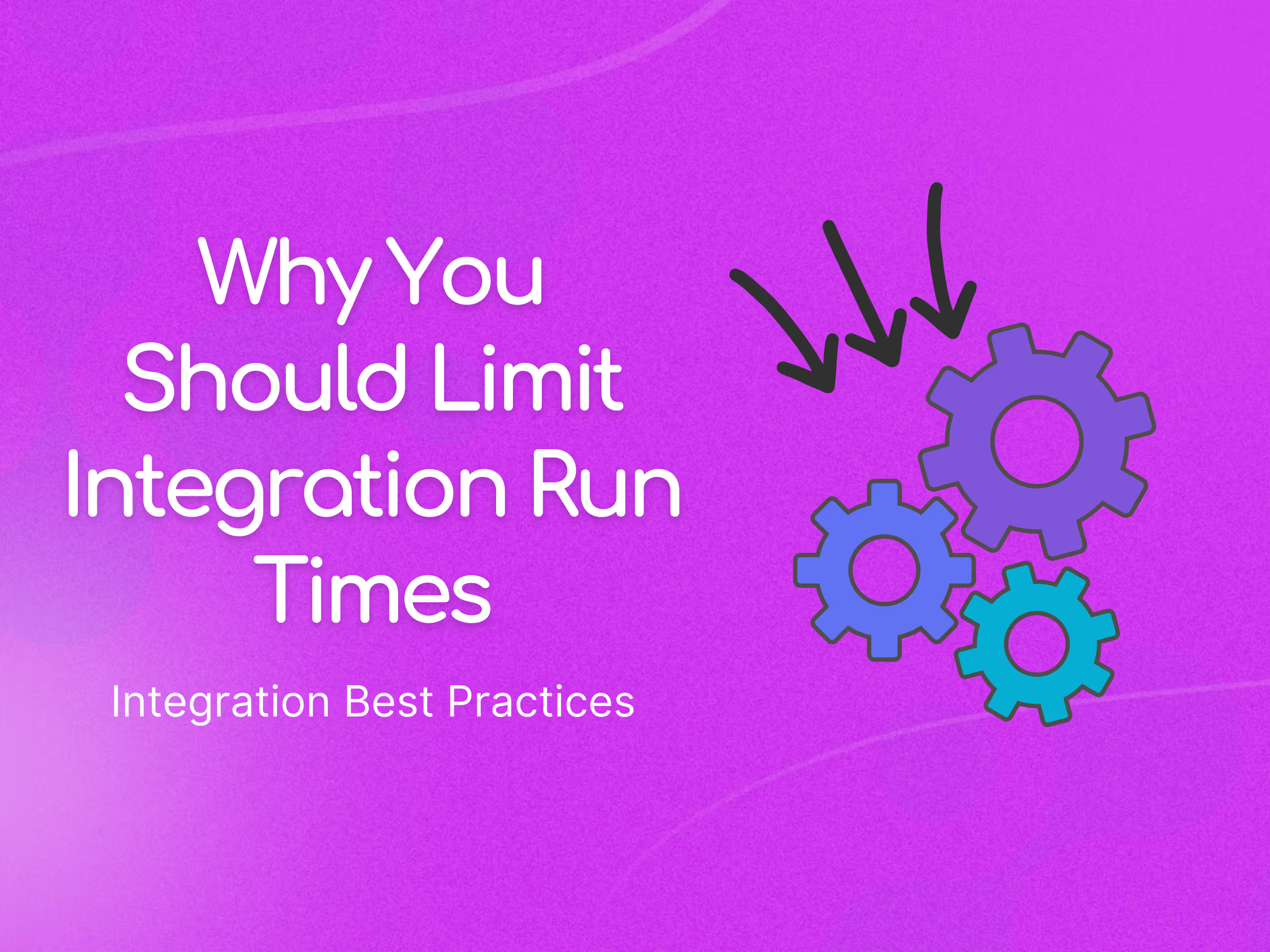
When you’re writing an integration between two APIs you generally have at least these three tasks:
- Fetch records from the first API.
- Transform each record in some way.
- Send the transformed record to the second API.
You should always consider how long the code will have to do that work. At Pandium every time we run an instance of an integration (which we call a tenant) we allow it to process for up to 10 minutes before ending it.
Some people find this surprising, so let’s unpack why.
Why you might think long/infinite run times will make your life easier
I routinely wish for more hours in each day, so I can relate to those who wish for more time in each run. There can often be several forces pushing us in that direction:
The integration has a lot of data to process
When more records need to be fetched, transformed, and sent off to the next system, it’s going to take more time. You can expect situations where your integration suddenly needs to handle a much larger volume of work:
- The first time a user runs the integration it may need to process a whole product catalogue or backfill data from 30 days ago.
- The API from which you’re pulling data may only upload records once a week in big batches.
- The integration needs to process far more orders during Black Friday.
One or more of the APIs is slow
Plenty of systems are affected by demanding API requests, resulting in suboptimal throughput due to network latency or their backend processing. For example, we’ve come across some well established APIs that can be like tractors; they’re powerful, and do everything, but they do it very slowly. Or perhaps you’re writing an integration for an early stage API that hasn’t had the opportunity to optimize everything yet.
Whatever the reason, you may often find your code waiting a long time for a response from an API. Allowing longer run times may seem like an easy way to compensate for that.
Why shorter runs are better
If each moment in an integration run is precious, then why do we voluntarily constrain ourselves to just 10 minutes? Because enforcing short run times will help you keep a tight ship.
Shorter runs encourage faster code
Users care about how quickly data is processed, so push yourself to make your integration efficient; limiting the length of a run can do that. It gives you an immediate reason to ensure that your local code runs as quickly as possible. It can also drive you to partner more closely with the developers of an API; if you find that a slow endpoint has become a key bottleneck for speed, you will be more likely to give useful feedback about it. That can help the API’s engineers address it, which will make your integration faster.
Shorter runs are easier to debug
If you are trying to find the details of a particular error, it can be overwhelming to dig through thousands of log lines in an infinitely long run. Shorter runs have a smaller number of logs, which are much easier to digest.
Shorter runs are more resilient
The longer a run is permitted to last, the greater chance it will encounter an error. This is even more true if you have a successful integration that has been installed by many users with high volume. Let’s explore what could happen when the integration encounters this inevitable error:
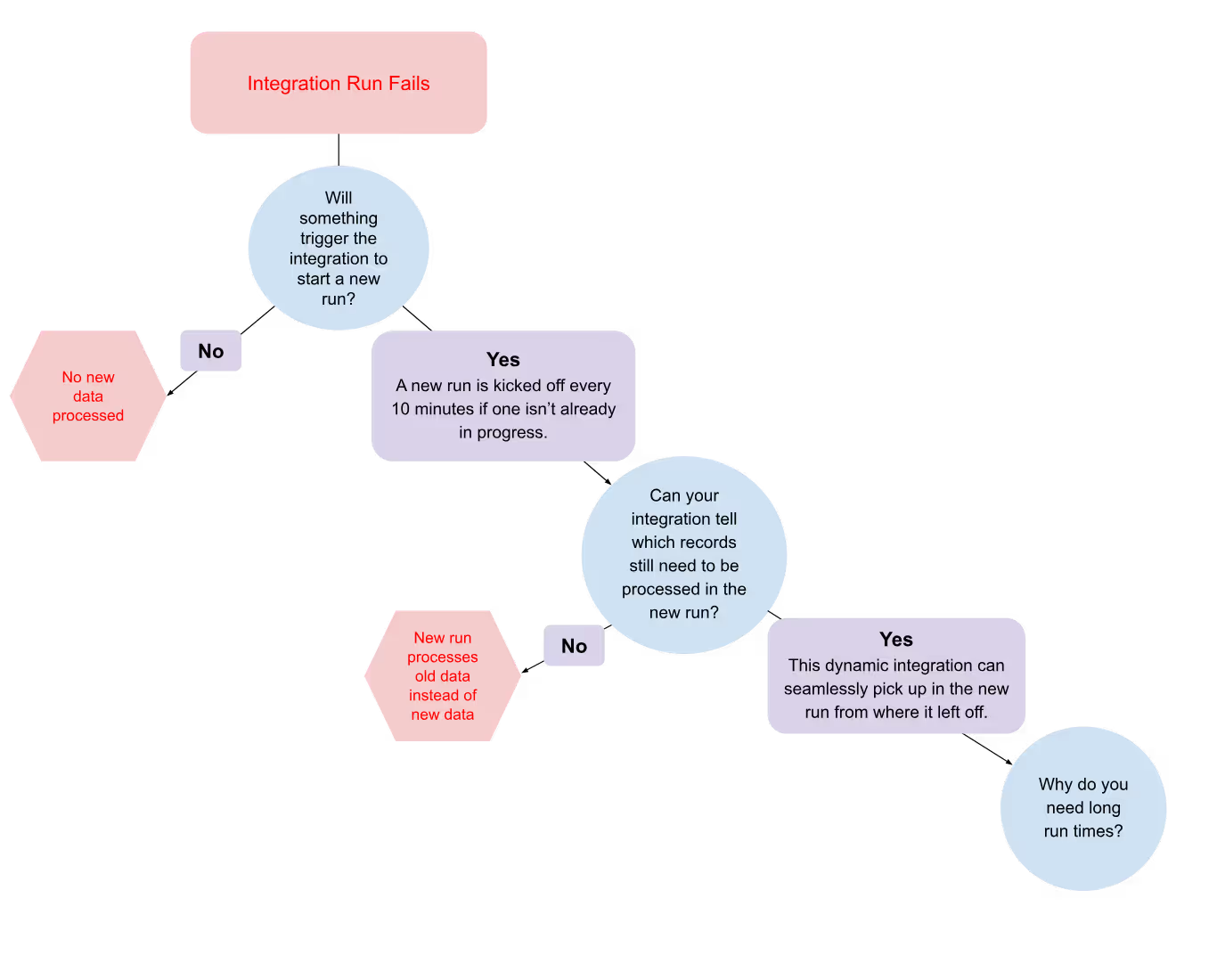
How to do everything you want with 10 minute runs
Here are some strategies we use to deal with the challenges of high data volume and slow APIs while still keeping run times under 10 minutes:
Use concurrency and parallelism when possible
These are great tools to make your code faster, and it’s a good way to work around an API that doesn’t have a bulk endpoint. This article goes into more detail about them.
Give the integration a way to determine which records from the first API were not already processed in a previous run.
Assume the integration will not be able to get through all the records available at the run’s start, so allow it to seamlessly continue work in the next run. When an integration is dynamic like this it doesn’t matter that each run is only 10 minutes long. The best way to make an integration dynamic will vary; this article covers different strategies that work for different APIs.
Don’t waste time processing records which the user may not care about
Your integration may be installed by many different users who usually want it to do slightly different things. Ask for that information when they install the integration, so you can help them.
Let’s look at an example of a hypothetical integration fetching customer records:

Different shops have different hopes for this integration:
- Some only care about Shopopolis customers who have placed an order in the past 3 months; they’re hoping to build loyalty in them.
- Others only want to send emails to customers who have not recently ordered; they want to bring them back.
When each user installs the integration, give them a settings page where they choose which customers should be imported. Use that information to add filters when fetching the Shopopolis customers.
Armed with these strategies, you give yourself the speed and resiliency of short runs while still dealing with the typical challenges of integrations.
From the Blog
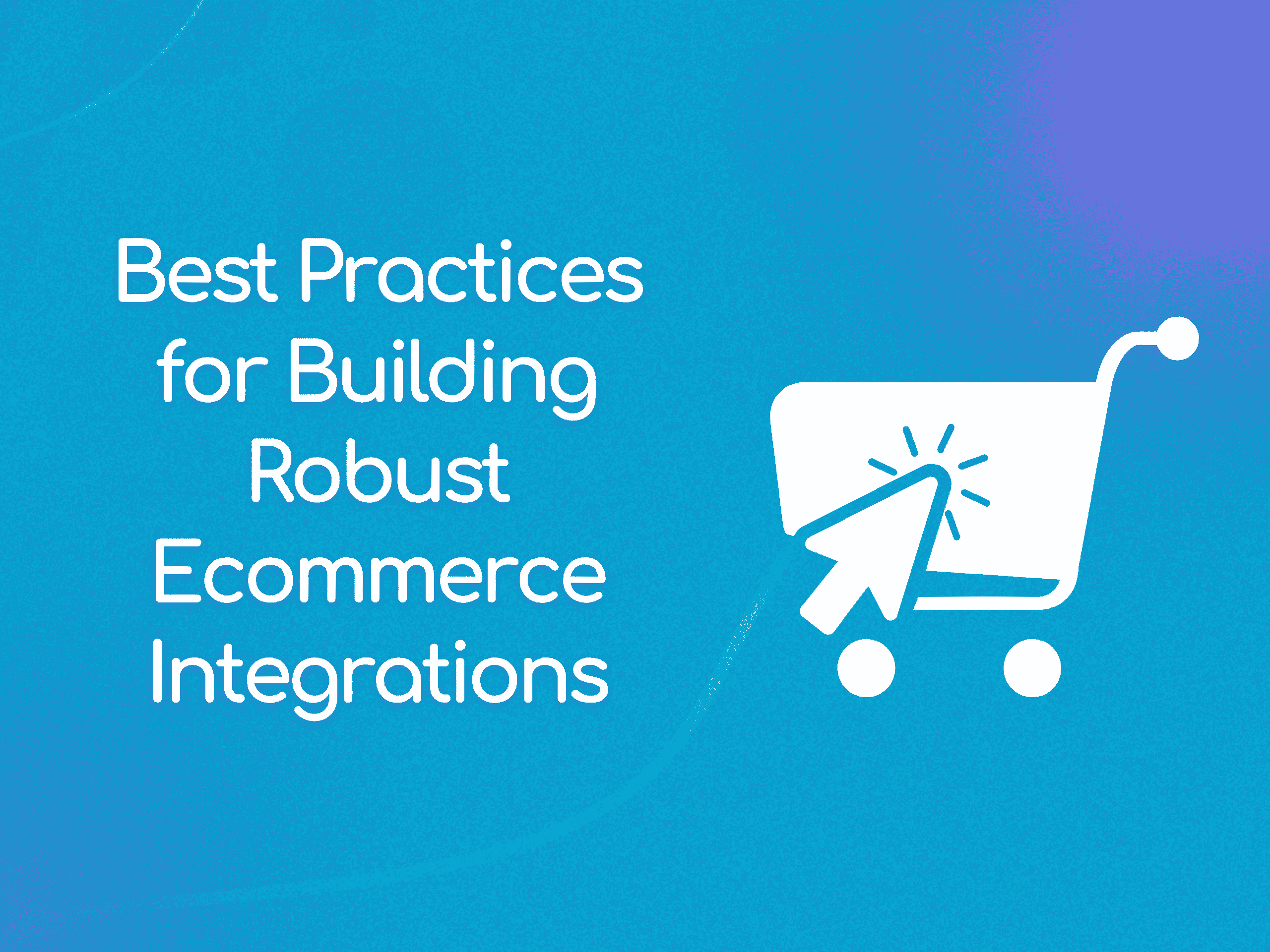
Best Practices for Building Robust Ecommerce Integrations
%20(1).avif)